Ask Addi P: How Can I Best Understand Predictive Analytics from My AI System?
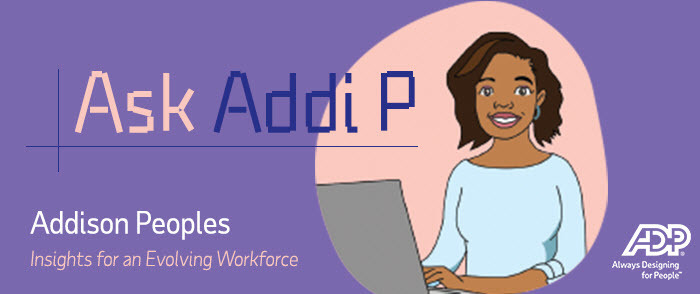
Dear Addi P,
Our organization just launched new AI based tools, including some with predictive analytics. How do I understand and use these predictions?
—Forecasting in Ft. Worth
Dear Forecasting,
This is such an important question. It's so easy to see a 70 or 80% prediction, assume it's a pretty sure thing and just go with whatever the prediction suggests. But whether or not you act on a prediction depends on what it's about, what the risks are and, most importantly, what else you need to know before you make the decision.
What are Predictions?
Predictions are statistical probabilities based on historical data and predictor knowledge. Some of the most common predictions we consider are weather forecasts. We can pretty accurately forecast the weather several days out, but it's also not unusual to walk outside with a 10% chance of rain, feel droplets and wonder what's going on. The forecast wasn't wrong, though — there was a chance of rain, and it rained.
It's important to remember that a prediction just tells you the statistical chances of something happening. The forecast may contain useful information, but reality isn't always going to conform to the prediction or your expectations.
What is the Risk?
Whether you follow a prediction should depend on what's at stake. If there's a 30% chance of rain, you might leave the umbrella behind because it's not the end of the world if you get a little wet. But if there's a 30% chance that your plane is going down, you wouldn't get on it. That's a life and death risk, so the tolerance for error — or even trusting the outcome to probabilities — is drastically reduced.
In HR technology, predictive analytics is being used to find the right candidates to interview, determine how someone might perform in a job, suggest career paths, forecast skills needed, and grant insights about turnover and how likely a person is to leave their job.
Let's look at flight risk. Suppose you get a prediction that Stephanie is 60% likely to leave in the next year. Perhaps your overall turnover rate is trending up and it looks like there will be greater attrition rates than usual for your department. At first glance, it may seem like you should schedule a stay interview with Stephanie to try to prevent her from leaving.
But what if Stephanie isn't performing that well? Or maybe the performance issues are a recent development — what should you do with this information then? If you tell Stephanie's manager she might be leaving, you run the risk of her manager treating her like she has already left, which can turn a prediction into a self-fulfilling prophesy. It's therefore important to consider the possible outcomes of any prediction before you make moves.
Ask More Questions
As you deal with predictions, keep the following key questions in mind:
- What do we want to happen? If Stephanie was probably going to be laid off or there's a headcount freeze and you want to put someone else on that team, maybe you do nothing and see what happens. But if you want Stephanie to stay, then you need to get more information about what's going on.
- What else do we need to know, and how do we find out? Maybe Stephanie's partner has been transferred to another city, or she has applied for another position within the organization. Perhaps she's dealing with health issues or a colleague is harassing her. These are matters you would need to know about to understand the situation fully, so see what information you can find out based on the records.
Typically, however, you will need to talk to Stephanie's manager, Stephanie herself or both before you make a decision. How you frame the discussion matters, and you should carefully weigh your outreach options against the probable outcomes so you can be prepared to handle them. If you need to talk to her manager, you might want to take a broader view and ask how the team is doing, who is most effective and what the team or department's needs look like from the manager's perspective. This can open the door to asking about individuals, including Stephanie.
Depending on what you learn, you can decide whether or not to follow up with Stephanie. If you do, be as transparent as possible so she understands that you are asking because you want her to stay, not because she is in trouble.
- What can we control? Once you have talked to Stephanie and you know what's going on, you have to figure out whether any challenges can realistically be addressed. If there are health issues, either personal or family-related, check with legal about FMLA protections. If there are problems with a co-worker or manager, get to the bottom of it and investigate any harassment concerns. If her partner is moving, find out where. Would it be possible to transfer her or offer remote work options? Bear in mind, you may not be able to do anything about the situation.
- What policies or exceptions apply? If you decide to offer a different schedule, remote work, time off or a raise, make sure those steps are consistent with your policies and that you are not only treating Stephanie fairly but also treating everyone else fairly in the course of finding a solution.
Predictive analytics can offer important and valuable information, but it is important to understand that even data-driven predictions are not crystal balls that foretell the future. What it can do, however, is offer new and insightful questions that lead to more effective decisions.
Take care,
Addi P.